Can AI take the place of a scientific writer?
- Farah Aladin-Foster
- Jul 10, 2024
- 7 min read
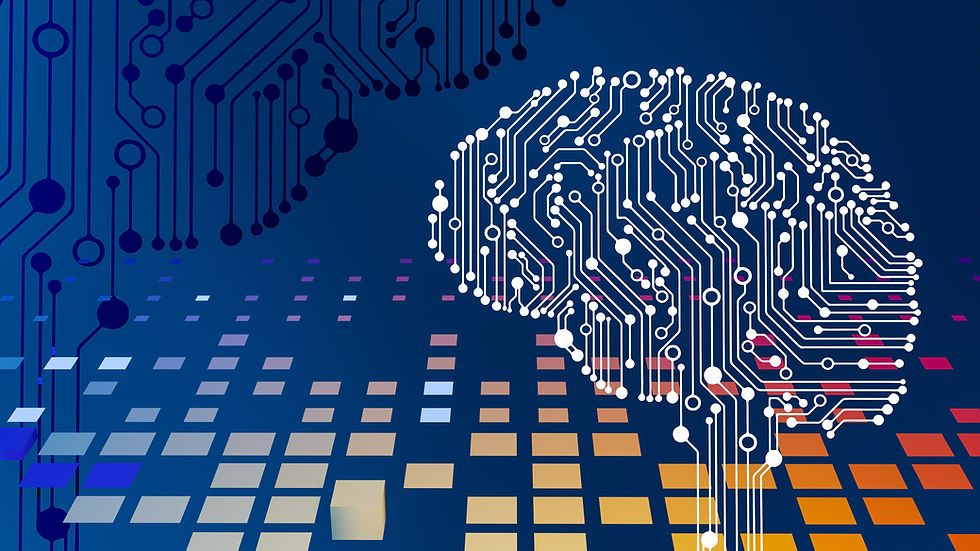
Artificial intelligence, or AI, is everywhere. There is no escaping it. You only need to look at the newly released smartphones to realise that what were once just the imaginings of science fiction writers, is now both accessible and affordable enough to carry in our pockets. The capabilities of AI are astonishing. It can write content, design art and even compose music. The past few years have seen a huge influx in both funding and research into this arena, and with the trajectory of the industry, the possibilities of just how far AI can develop even in the immediate future, really do seem unlimited.
But my question here is, how useful (or even relevant), is the use of AI in scientific writing? We know that AI can generate content on almost any subject imaginable, but in terms of us who spend our lives detailing scientific concepts, with accuracy and precision at the heart of all we create, can AI really supersede our roles?
I am no software engineer, nor will I even attempt to describe the complexities of coding such technology – I will leave that firmly in the hands of the experts. However, through hands-on experience I feel I can say I have at least a basic understanding of AI and its application in scientific writing, so here is my take on where I see this technology now, and its potential scope for the future.
There has been an emergence of two forms of AI, both of which leverage the power of machine learning. They have opposing foundations in the principles and development of their training module algorithms, the subsequent handling of data and finally the generation of results. Before we can discuss how we can use AI in our writing careers, it is important to be familiar with these different AI frameworks.
Predictive vs generative AI
Predictive AI is based on the AI algorithm analysing pre-existing data, and generating content from what it reviews. This could be searching a compiled database or reviewing legacy information. This means that should no appropriate data be present, then the algorithms would either fail to return any relevant outputs, or create hallucinations which are either meaningless, incorrect or out of scope. Users of predictive AI tend to be those that rely on historic data to predict future outcomes, including performing market forecasts or seeking trends in customer behaviour, where analysis needs to be wholly dependent on the dataset. While there are benefits of knowing exactly where the information is being sourced from, predictive AI is not without issues. There is the risk of overfitting, where the algorithm forces a result due to a lack of substantiated data, or if the database contains high complexity information, the AI could struggle to fully grasp the nuances of data and present misinterpreted results.
Generative AI is the creation of completely novel content based purely on the input information. Unlike predictive AI, there is no pre-existing knowledge stored within the AI module, so the outputs can be incredibly creative but are prone to increased error as there is no reference database by which to align to. This means that generative AI tends to be used by those in the creative industries, including music and art, where flexibility is encouraged to drive the brief. One of the main limitations, especially for generative AI platforms that are designed with autoregressive algorithms (where each new iteration is a refinement based on the results of the previous outcome), is that misinformation can become baked into the outputs. This continued reliance on an incorrect result can make it difficult for the algorithms to move on to an alternative pathway, and so the outcomes can become more obscure with increasing deviation from the brief.
Scientific writing appears to fall somewhere between both predictive and generative AI models. More formal writing (e.g. academic papers and grant proposals) would lean towards predictive AI, where the outputs should be ultimately dependent on a foundation of detailed and accurate science. In comparison, blog posts and informal web content fit better into the generative AI model, where creativity and novel content is required to make the writing stand out. So, while all formats of scientific writing require a solid grounding in order maintain precision and scientific plausibility, there is clearly a need for creativity and to look at information in a more open-minded manner to factor in concepts and relationships perhaps not necessarily previously considered.
My experiences with AI
I was lucky enough to have worked on an AI development project in the role of a scientific writer. The aim of the project was to use AI to aid with technical queries from customers, and I led a team responsible for providing guidance to the developers and for ensuring the accuracy of the returned answers. The platform was initially developed using a predictive AI model, with the aim to move to a more generative AI algorithm as the machine learning continued to adapt and learn over time.
The preliminary versions of the predictive AI software worked well, even accurately extrapolating the scientific principles to expand on the answer. The tone of the language was more informal than desired, but we understood that this would adapt over time through machine learning. However, if there was no scientific context present from which to refer from, the answers generated made grammatical sense, but had no scientific relevance to the topic. I will admit, we only had a limited dataset to work with, so I believe the true test would have been to refer to information in different formats from a larger, more comprehensive database and then assess how the AI coped in delivering the desired output. Despite this limited testing environment, there was clear potential. Even in the handling of complex scientific concepts, the predictive AI module appeared to work well when there was reference data to rely on.
Using AI as a scientific writer
In contrast to using AI in generating highly technical customer responses, I have also used AI to generate scientific content for a communications brief. However, for this more informal writing project, I had to spend a considerable amount of time on a rewrite. Firstly, I had to adjust the tone of the language to meet the brief. Secondly, I was unconvinced of many of scientific claims, and so I had to spend time fact-checking and adjusting the context of certain sentences to ensure that the science was not lost in translation. And finally, because the content was AI generated, I had to reformat the piece to sure make the writing was unique. In my opinion, it would have been easier to do my own research and write the piece from scratch. However, it was not all negative. The AI platform I used threw a few interesting nuggets of information I had not considered including, so I am thankful to the algorithm for pulling those forward for me to incorporate into the brief.
What I found useful when using AI
Providing inspiration for a starting point – As a writer, sometimes there is nothing scarier than a blank page, especially when starting to work on a topic which is more unfamiliar than normal. Using AI can provide a good place to start, and to focus your thinking along the right tracks.
Illustrating examples of structure and format – Even in the traditional world of academic journals, not even considering more informal marketing collateral formats, there are a multitude of structure types which scientific content can follow. AI can be used to pull forward ideas of the most appropriate formats for your writing brief.
Generating ideas not necessarily considered – One of the beauties of AI is that is it can be creative in the most unexpected places. While this might not be ideal for maintaining scientific accuracy, it can generate ideas which you may not have considered, adding a level of depth to your content.
Areas to be taken under consideration with AI
Content may be pulled word-for-word from other sources – Care should be taken to ensure that you are not in breach of any plagiarism regulations. AI could copy language verbatim from another source without any reference information, leading to the possibility of plagiarism.
Generated content may not be novel – AI algorithms, though creative, are still regulated by rules. Repeatedly asking the same question into the same AI platform will, unsurprisingly, generate the same output. Should another writer be using the same platform and ask the same question, then we run the risk of duplicated content. One of advantages of being a writer is the flexibility and creativity we can inject into our work. AI has no such luxury when bound by the rules of their algorithms, especially in a predictive AI model.
Not all the information will be correct – While AI is getting more intelligent with each new development, it can still run into issues with fact-checking. The AI algorithms will ensure that the grammar and syntax are correct, but the science? This will not always be the case. It is therefore important to always have a “human in the loop” and not take the AI-generated science as gospel.
A final note…
AI is here to stay, whether we like it or not. There is no putting the genie back in the bottle. The technology clearly has potential, and this area of research seems to have no bounds. It is only a matter of time before a brand-new function emerges, and what we see as groundbreaking and ingenious today will soon seem archaic and outdated. And coming back to the topic at hand, is there room for using AI for scientific writing? There is immense possibility. It needs honing, polishing and refining (and many other similar adjectives), but if we can teach these algorithms to be both structured yet flexible with the content, then who knows what new outputs these platforms can create. So, while I am excited to see what the future holds, I am also secretly relieved that (for now at least) there is still a need for us scientific writers to do what we do best, and we are yet to be replaced by machines.
Comments